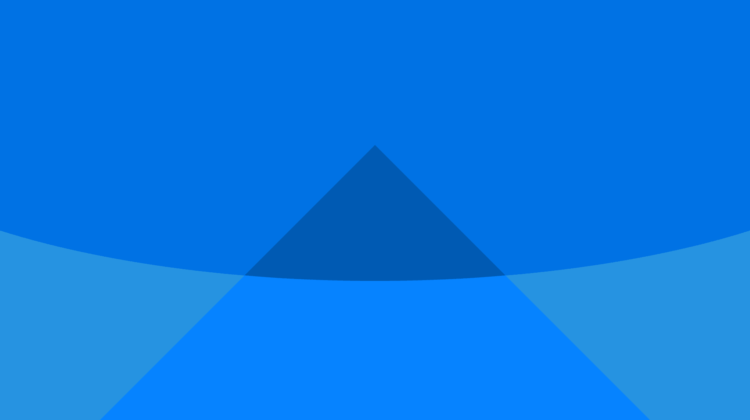
Global Economy: Predictive Models and Their Impact
In the realm of modern economics, predictive models have become indispensable tools for policymakers, financial analysts, and business leaders. These models, driven by advanced algorithms and vast datasets, aim to forecast economic trends, anticipate market fluctuations, and guide strategic decision-making. The impact of predictive models on the global economy is profound, influencing everything from monetary policy and investment strategies to supply chain management and consumer behaviour. However, the increasing reliance on these models also raises critical questions about their accuracy, transparency, and ethical implications. This article delves into the intricacies of predictive models in the global economy, examining their development, application, and the multifaceted impact they exert on various economic sectors.
The evolution of predictive models in economics is a testament to the rapid advancements in computational technology and data science. Historically, economic forecasting relied heavily on qualitative analysis and relatively simplistic quantitative methods. Early models, such as those developed by Jan Tinbergen and Lawrence Klein, laid the groundwork for modern econometrics but were constrained by limited computational power and data availability. The advent of powerful computers and the proliferation of big data have revolutionised this field, enabling the development of sophisticated models that can process vast amounts of information and uncover complex patterns. Machine learning algorithms, in particular, have become central to predictive modelling, offering enhanced accuracy and the ability to adapt to new data.
The applications of predictive models in the global economy are extensive and varied. In monetary policy, central banks utilise these models to forecast inflation, employment rates, and GDP growth. By analysing historical data and economic indicators, predictive models help policymakers anticipate economic conditions and make informed decisions about interest rates and other monetary tools. For instance, the Federal Reserve’s use of dynamic stochastic general equilibrium (DSGE) models illustrates how predictive analytics can guide policy interventions aimed at stabilising the economy. Similarly, the European Central Bank employs predictive models to assess the impact of unconventional monetary policies, such as quantitative easing, on the eurozone economy.
In the financial sector, predictive models are integral to risk management and investment strategies. Hedge funds and asset managers leverage quantitative models to predict market movements, identify investment opportunities, and optimise portfolio performance. High-frequency trading firms, in particular, rely on predictive algorithms to execute trades at millisecond speeds, capitalising on minute price discrepancies. The proliferation of artificial intelligence in finance has further enhanced the predictive capabilities of these models, enabling more sophisticated analysis of market sentiment and macroeconomic trends. However, the increasing complexity of financial models also raises concerns about systemic risk and market volatility, as evidenced by the role of algorithmic trading in events like the 2010 Flash Crash.
Beyond monetary policy and finance, predictive models have significant implications for global supply chains and production processes. Companies use these models to forecast demand, optimise inventory levels, and streamline logistics. The ability to predict consumer behaviour and market trends allows businesses to reduce costs, improve efficiency, and enhance customer satisfaction. For example, predictive analytics in retail can anticipate shifts in consumer preferences, enabling companies to adjust their product offerings and marketing strategies accordingly. In manufacturing, predictive maintenance models help prevent equipment failures and minimise downtime, contributing to more resilient and efficient production systems.
The impact of predictive models extends to the labour market and workforce planning as well. Employers use predictive analytics to identify skill gaps, forecast hiring needs, and develop training programmes. By analysing trends in employment data, predictive models can inform policies aimed at addressing unemployment and underemployment. For instance, during the COVID-19 pandemic, predictive models helped governments and organisations assess the impact of lockdowns on labour markets and design interventions to support affected workers. Moreover, predictive models in education can identify at-risk students and tailor interventions to improve academic outcomes, thereby enhancing the alignment between education systems and labour market demands.
Despite the numerous benefits of predictive models, their increasing use also poses significant challenges and ethical considerations. One of the primary concerns is the accuracy and reliability of these models. Predictive models are only as good as the data they are based on and the assumptions they incorporate. Inaccurate or biased data can lead to flawed predictions and misguided decisions. For instance, the failure of many predictive models to foresee the 2008 financial crisis highlighted the limitations of existing economic models and the need for more robust and transparent methodologies. Moreover, the black-box nature of some advanced algorithms, particularly in machine learning, raises concerns about accountability and interpretability. Policymakers and stakeholders may find it difficult to understand how predictions are generated, complicating efforts to evaluate and trust the model’s recommendations.
Ethical considerations are also paramount in the use of predictive models. The potential for discrimination and bias in algorithmic predictions is a significant issue, particularly when models are used in areas such as hiring, lending, and law enforcement. Biased algorithms can perpetuate existing inequalities and result in unfair treatment of individuals based on race, gender, or socioeconomic status. Ensuring fairness and transparency in predictive modelling requires rigorous testing, validation, and oversight. Additionally, the use of personal data in predictive models raises privacy concerns. The vast amounts of data collected and analysed by companies and governments must be handled with care to protect individuals’ privacy rights and maintain public trust.
Furthermore, the globalisation of predictive models necessitates international cooperation and standardisation. Economic predictions often have cross-border implications, affecting global trade, investment flows, and geopolitical stability. Developing international standards for data sharing, model validation, and ethical practices can help harmonise predictive modelling efforts and mitigate the risks associated with disparate regulatory environments. Collaborative initiatives, such as the OECD’s work on artificial intelligence and predictive analytics, illustrate the importance of global cooperation in addressing the challenges and opportunities of predictive models in the global economy.
In conclusion, predictive models have become a cornerstone of modern economic analysis and decision-making, offering powerful tools to forecast trends, manage risks, and optimise performance across various sectors. Their impact on the global economy is profound, influencing monetary policy, financial markets, supply chains, and labour markets. However, the increasing reliance on predictive models also brings significant challenges related to accuracy, transparency, ethics, and international cooperation. Addressing these challenges requires a multidisciplinary approach, integrating insights from economics, data science, ethics, and policy. As predictive models continue to evolve and shape the global economy, ensuring their responsible and equitable use will be crucial in harnessing their full potential for sustainable and inclusive growth.
Author: Harvey Graham
Forecast analysis consultant in Great Britain. Collaborates with The Deeping in the economic forecasting area